For imaging centers, efficiently scheduling patients for MRI, CT, and PET scans impacts revenue, patient satisfaction, and the ability to accommodate urgent cases.
Yet juggling available time slots across devices, technologists, and radiologists while considering patient needs and priorities becomes highly complex, especially at extensive, busy facilities.
Add perpetually changing variables like last-minute emergencies or late arrivals, and this balancing act easily topples.
Luckily, artificial intelligence (AI) innovations offer advanced solutions for optimizing scheduling through the RIS radiology information system.
The High Costs of Status Quo Scheduling
Most facilities schedule scans via traditional RIS tools, which enable coordinators to manually assign patient appointments based on device and staff availability.
However, these solutions fail to account for numerous interdependent constraints impacting optimal throughput. Consequences include:
- Long patient wait times lower satisfaction
- Idle but unavailable slots reduce scan volume
- Scrambling to accommodate urgent cases increases staff stress
- Overbooking and patient backlogs from guessing demand
When operating below maximum efficiency, the budget suffers through reduced reimbursements, as fixed costs stay stagnant as revenue-generating scan volume lags.
AI-powered scheduling eliminates these pain points through intelligent automation.
How AI RIS Scheduling Works?
AI scheduling is powered by deep reinforcement learning algorithms trained on facility-specific data, including:
- Historical procedure types/duration: To forecast resource needs
- Patient clinical data: To estimate scan durations and contrast use
- Staff/device availability: Considering real-time changes
- Department KPIs: Managing constraints like budget goals
By accounting for these interdependent dynamics, AI can optimally schedule patients for high priority and throughput while meeting clinical needs.
Key capabilities enabled include:
- Realignment recommendations: Suggests appointment changes increasing resource utilization when disruptions occur
- Priority-based bookings: Reserves capacity for urgent cases while safely overbooking electives per tolerance levels
- Risk scoring: Flags and prevents unsafe overbooking scenarios
- What-if planning: Models appointment scenarios considering constraints
- Wait time reduction: Decreases patient cycle times and backlogs
- KPI impact forecasting: Predicts scheduling decisions effects on budgets
As algorithms ingest more departmental data, scheduling recommendations continuously improve.
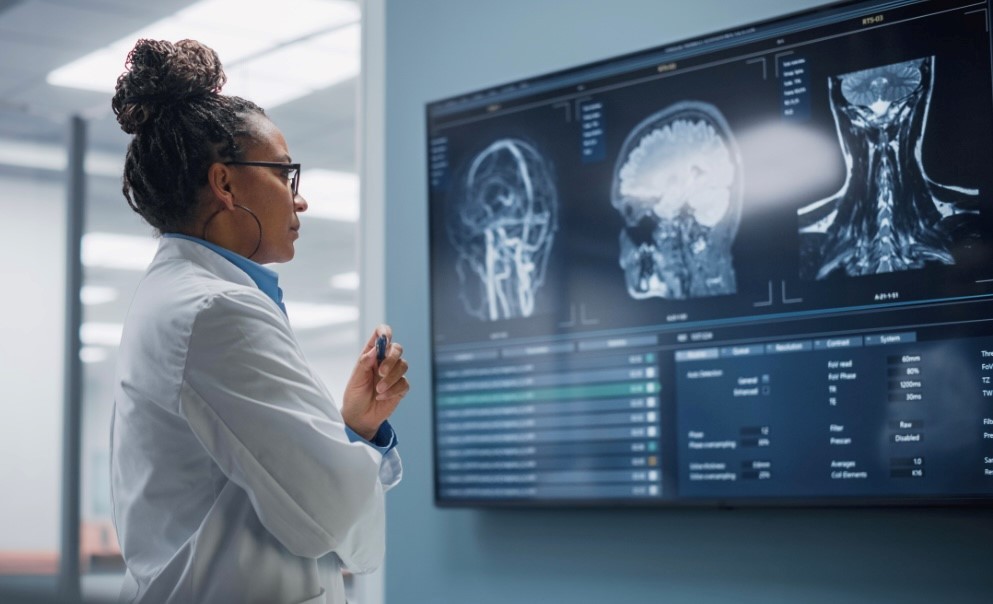
Staff Roles and Perceptions
Some radiology staff initially view AI scheduling suggestions skeptically, worrying automation supplants human judgment or control.
In practice,, though, AI augments coordinators’ efficiency, handles tedious number crunching,, and provides data-driven input to aid better decisions.
Staff responsibilities transforming include:
- Scheduling coordinators: Still handle patient communications but rely more on algorithm-generated slot suggestions. Focus shifts from manually juggling schedules to higher-value tasks like customer service.
- Technologists: Experience reduced frustration from last-minute appointment shuffling. AI considers real-time resource constraints.
- Department heads: Analytics dashboards provide insight into schedule optimization effects on KPIs like budget metrics. Allows data-driven management.
Implementation Considerations
While AI RIS scheduling drives significant efficiency gains, obtaining full value requires adjusting existing protocols, including:
- Historical data quality: Systems benefit from clean, normalized inputs over longer time horizons. Improving legacy data practices may be necessary.
- Staff adoption: Leadership should foster a culture of welcoming optimization algorithms that assist human schedulers rather than replace them outright.
- Cyber security: Cloud connectivity introduces vulnerabilities requiring special safeguards protecting patient data and systems.
With planning and stakeholder onboarding, facilities avoid pitfalls and leverage the full potential of AI scheduling technologies alongside staff coordinators.
Radiology centers enhance resource utilization, increase patient volumes, and protect bottom lines through revolutionized scheduling practices by harnessing AI tools that seamlessly integrate with traditional RIS platforms.
Furthermore, staff can focus on high-value tasks like patient relations rather than manually juggling endless appointment variables.
With the right vision and preparation for associated growing pains, innovative AI integration promises smarter, faster, and more responsive scheduling, unlocking imaging center productivity in the years ahead.